It seems to be an increasingly rare occurrence that you can browse an online shopping website or look for a new mobile phone deal and not be distracted by a small pop-up box in the bottom corner of the screen with “someone” asking if they can help. Artificial Intelligence (AI) chatbots are commonplace; in fact, they are often the primary (and sometimes preferred) way in which we communicate with companies in many consumer industries. But what about the role of AI in the form of chatbots and beyond in life sciences?
AI is introducing efficiencies and opportunities for innovation across functions within pharma, from optimising communication with patients and key physician stakeholders to supporting new ways to run R&D and effectively tackle data analytics.
AI Chatbots and Machine Learning
There has already been a move towards virtual healthcare from providers and insurance companies through video consultations and messaging services to initiate the patient journey. The convenience and timely provision of advice allowed by this approach evidently resonates with customers and represents an opportunity to improve how pharma companies communicate with patients and physicians alike.
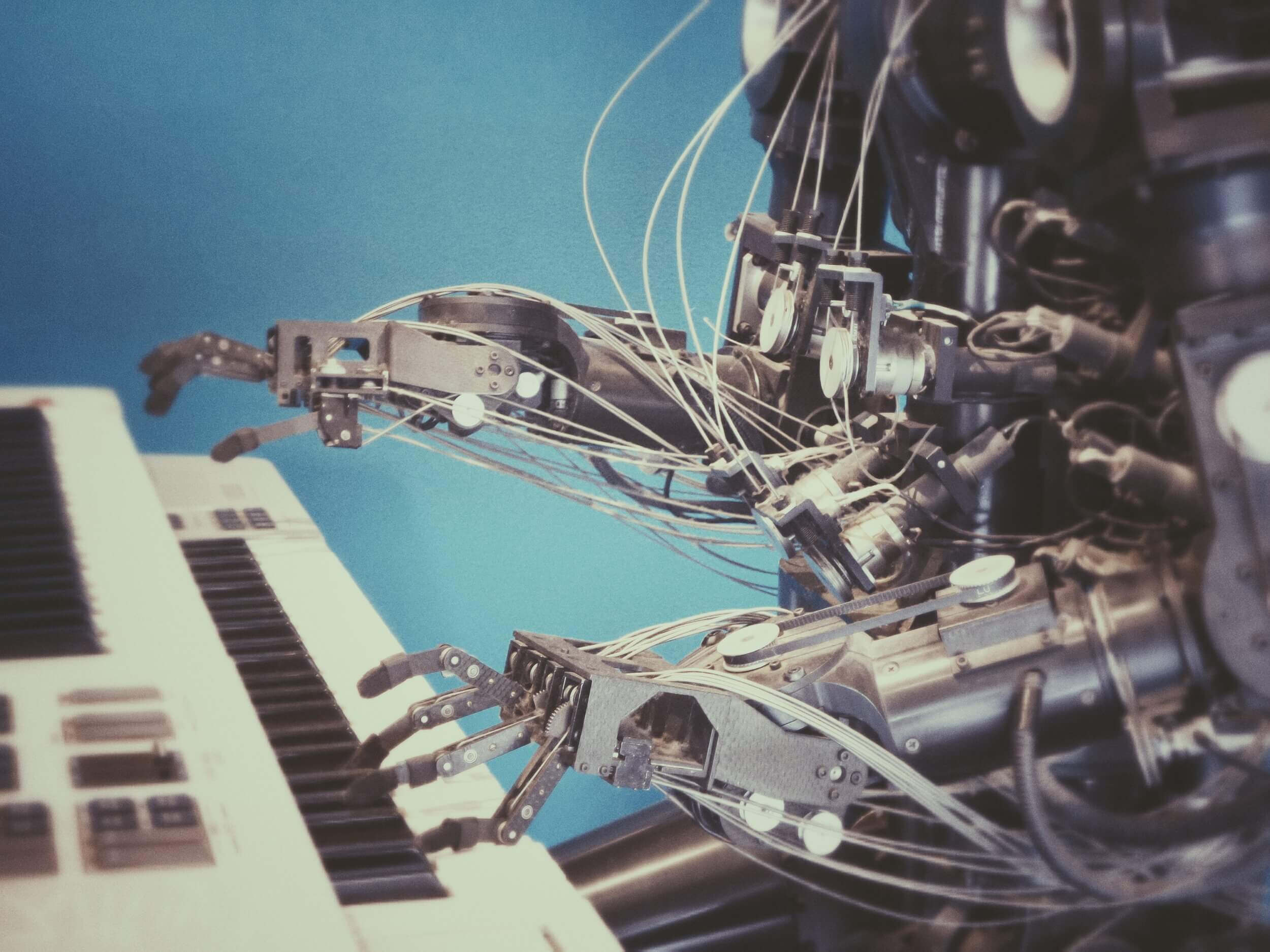
Introducing AI chatbots into the fold can allow for communication with patients to answer questions, provide further information on therapies, prompt treatment reminders and provide support with any complex administration schedules/procedures. Additionally, these tools can be used to support adverse event/non-compliance reporting (depending on local regulations). Essentially, by communicating with patients in a more efficient way, we can provide a better service to support the management of their disease, potentially leading to improved outcomes.
Another avenue to consider is the potential to improve interactions with healthcare providers (HCPs). From a customer service and sales function angle, chatbots built into broader relationship management systems can ease the administrative burden of ensuring that meetings with HCPs are scheduled, reminders set up, and sample orders placed. Less time spent on repetitive tasks enables more time for sales reps to focus on having meaningful conversations with these stakeholders. What about the decision around which HCPs to engage? AI can also support the development of an HCP targeting strategy, with potential for continued machine learning to assess the true impact of various stakeholder interactions. Understanding the impact of what we say and who we say it to could be extremely valuable in optimising these engagements.
Drug Discovery
AI and machine learning are also increasingly utilised by pharma companies in shaping how they develop new therapies and diagnostic tools. One example can be seen with Oxford-based AI start-up Exscientia, which entered the first entirely AI-invented drug molecule into human clinical trials in January 2020, just 12 months after the programme began. Additionally, as part of a joint venture, the company is putting its technology to use to screen almost every known approved and investigational drug (~15,000 molecules) against drug targets for COVID-19 in efforts to accelerate the development of a treatment. With use of these AI drug discovery platforms steadily increasing, we can expect to see a dramatic shift in the time it takes to get a drug candidate into clinical trials.
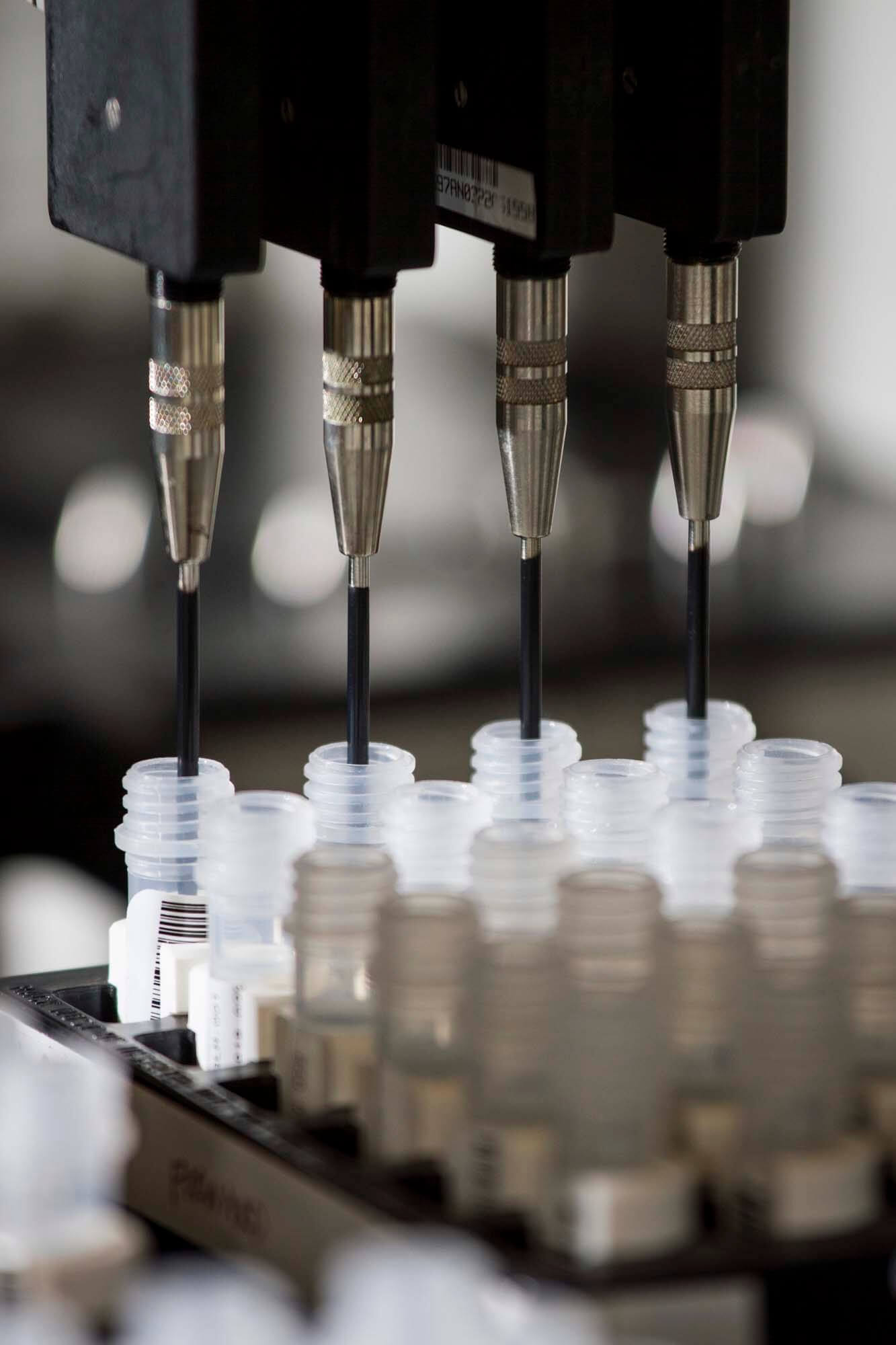
Data Analytics
Finally, how can we leverage AI to understand how our medicines work outside of the clinical trial setting? There is already consensus around the significant value of real-world evidence, but the value of this data can be further amplified using AI. The ability to mine large, real-world datasets can enable us to draw compelling insights around the daily burden of disease or even uncover new patient sub-populations.
Beyond the traditionally available real-world data, data around behavioural measures and environment can play a substantial role in understanding how a patient may respond to a treatment and, in some cases, even predict disease. An example of players with these capabilities include Evidation Health, a technology and services company that leverages machine learning to pinpoint these factors and create a set of ‘digital biomarkers’ that can be used to guide treatment and predict onset of a disease (e.g. by articulating the link between breathing abnormalities and a serious asthma attack). Through partnerships with such service providers, pharma companies can ensure a better understanding of daily disease burden and, in response, develop solutions that substantially improve patient outcomes.
From R&D to the mining of big data to optimise use of a therapy, AI’s role is increasing across the many facets of the product lifecycle. When thinking about such an extended timeframe, two critical success factors stand out for ensuring the long-term success of a brand: having knowledge of where the market is going and being creative with internal and external solutions. AI-based solutions can contribute to both of these factors, with AI itself being a creative solution that can inform the brand team of the real unmet needs for a disease or with a product and facilitating the opportunity to drive innovation that truly addresses the market needs.
For more insights on how to achieve long-term success for a brand or portfolio (and to ensure our strategy taps into and maximises relevant new advances in the industry), stay tuned for our upcoming series, spanning early to late strategy decision points within Lifecycle Management.
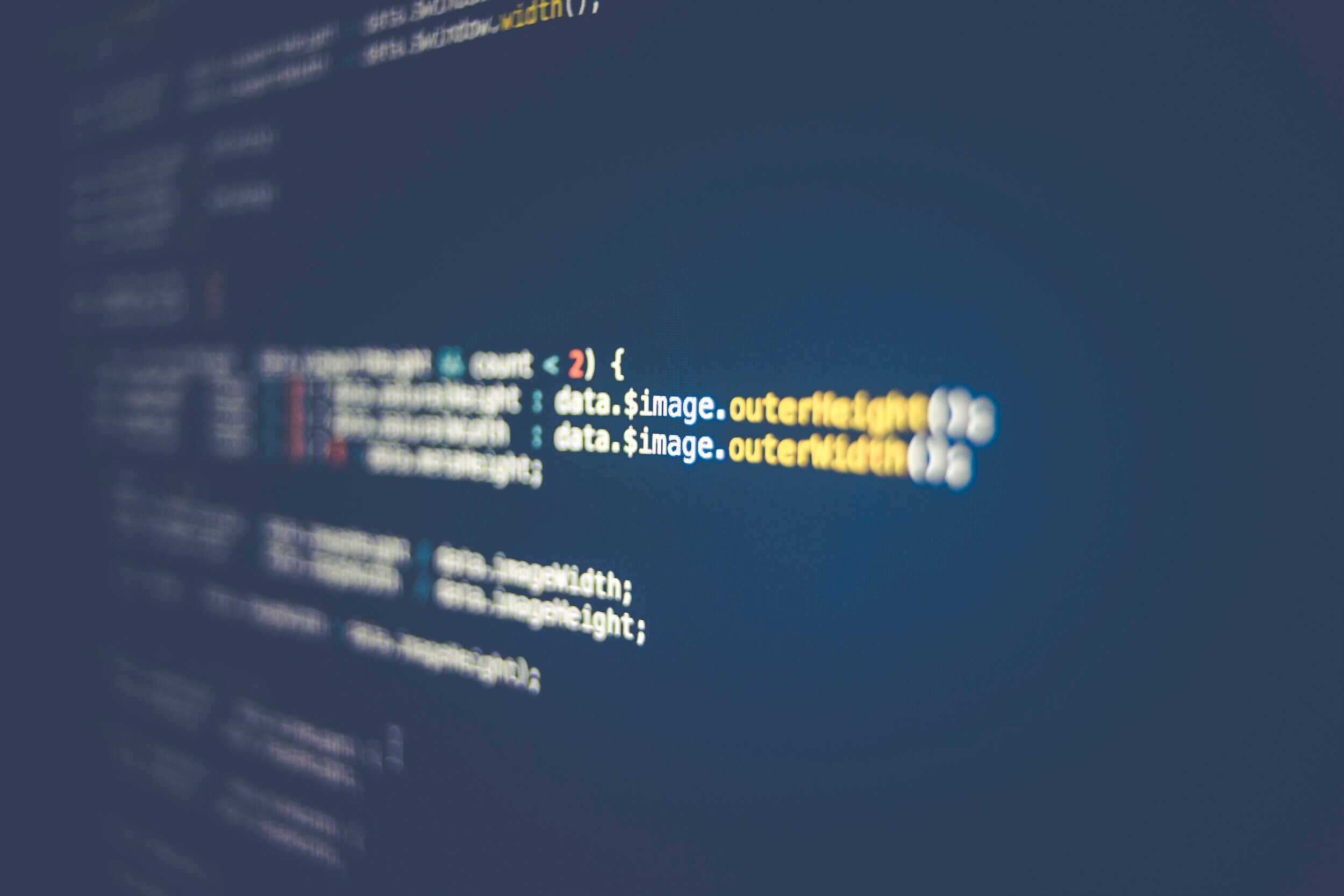